Multimodal output opens up new prospects
Having true multimodal output opens up attention-grabbing new prospects in chatbots. For instance, Gemini 2.0 Flash can play interactive graphical video games or generate tales with constant illustrations, sustaining character and setting continuity all through a number of photos. It is from excellent, however character consistency is a brand new functionality in AI assistants. We tried it out and it was fairly wild—particularly when it generated a view of a photograph we supplied from one other angle.
Making a multi-image story with Gemini 2.0 Flash, half 1.
Google / Benj Edwards
Making a multi-image story with Gemini 2.0 Flash, half 2. Discover the choice angle of the unique photograph.
Google / Benj Edwards
Making a multi-image story with Gemini 2.0 Flash, half 3.
Google / Benj Edwards
Textual content rendering represents one other potential power of the mannequin. Google claims that inner benchmarks present Gemini 2.0 Flash performs higher than “main aggressive fashions” when producing photos containing textual content, making it doubtlessly appropriate for creating content material with built-in textual content. From our expertise, the outcomes weren’t that thrilling, however they had been legible.
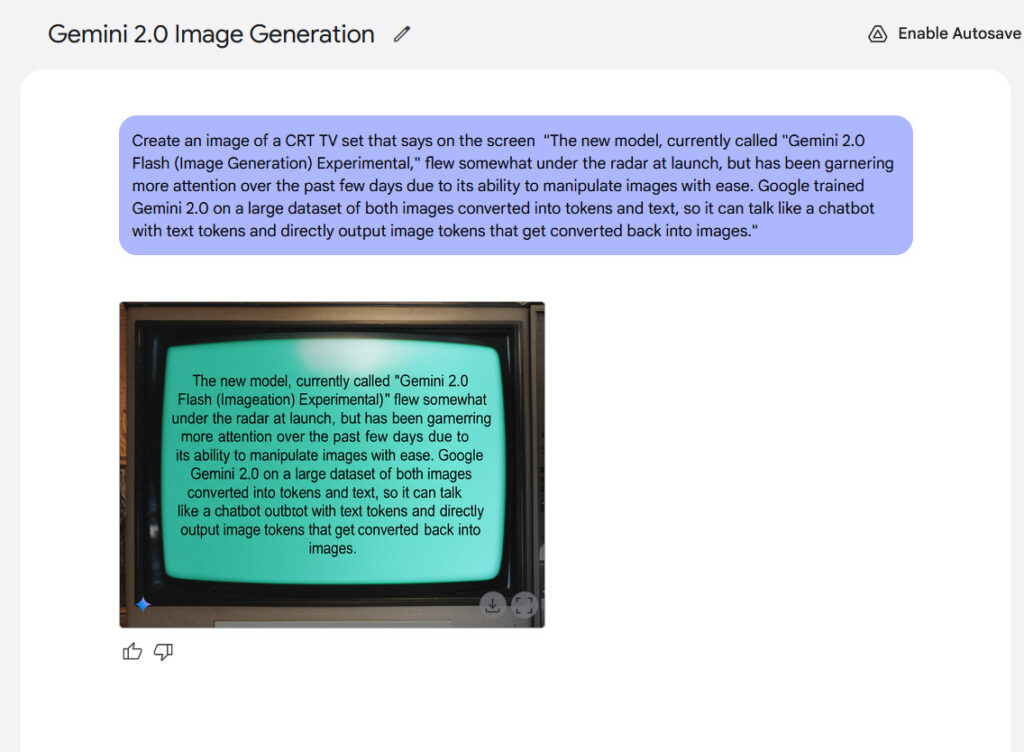
An instance of in-image textual content rendering generated with Gemini 2.0 Flash.
Credit score:
Google / Ars Technica
Regardless of Gemini 2.0 Flash’s shortcomings to date, the emergence of true multimodal picture output appears like a notable second in AI historical past due to what it suggests if the know-how continues to enhance. In the event you think about a future, say 10 years from now, the place a sufficiently complicated AI mannequin may generate any sort of media in actual time—textual content, photos, audio, video, 3D graphics, 3D-printed bodily objects, and interactive experiences—you mainly have a holodeck, however with out the matter replication.
Coming again to actuality, it is nonetheless “early days” for multimodal picture output, and Google acknowledges that. Recall that Flash 2.0 is meant to be a smaller AI mannequin that’s sooner and cheaper to run, so it hasn’t absorbed your entire breadth of the Web. All that data takes a whole lot of area by way of parameter depend, and extra parameters means extra compute. As a substitute, Google educated Gemini 2.0 Flash by feeding it a curated dataset that additionally possible included focused artificial knowledge. Consequently, the mannequin doesn’t “know” the whole lot visible in regards to the world, and Google itself says the coaching knowledge is “broad and normal, not absolute or full.”
That is only a fancy manner of claiming that the picture output high quality is not excellent—but. However there may be loads of room for enchancment sooner or later to include extra visible “data” as coaching strategies advance and compute drops in price. If the method turns into something like we have seen with diffusion-based AI picture turbines like Steady Diffusion, Midjourney, and Flux, multimodal picture output high quality could enhance quickly over a brief time frame. Prepare for a totally fluid media actuality.